Holt Winters Example
Holt Winters filter was mentioned in the comments. It's a popular choice for smoothing and forecasting certain kinds of seasonal series, and it can deal with nonstationary series. Particularly, it can handle series where the mean level grows linearly with time. In other words where the slope is stable. In my terminology the slope is one of the invariants that this approach extracts from the series. Let's see how it fails when the slope is unstable.
In this plot I'm showing the deterministic series with exponential growth and additive seasonality. In other words, the slope keeps getting steeper with time:
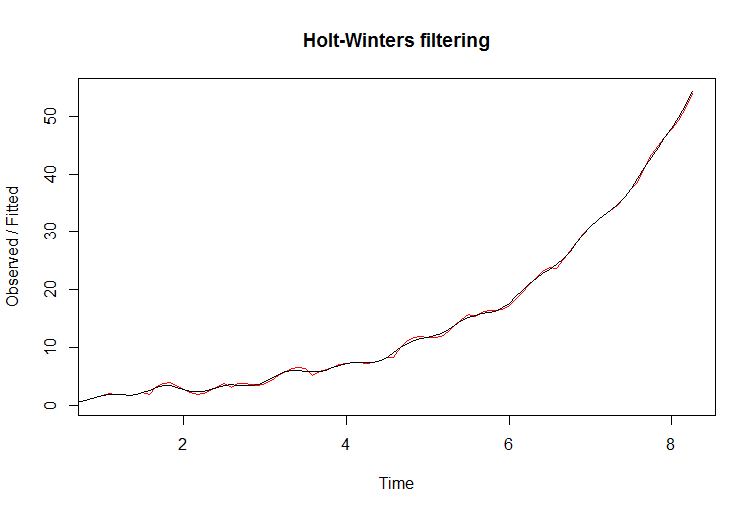
You can see how filter seems to fit the data very well. The fitted line is red. However, if you attempt to predict with this filter, it fails miserably. The true line is black, and the red if fitted with blue confidence bounds on the next plot:
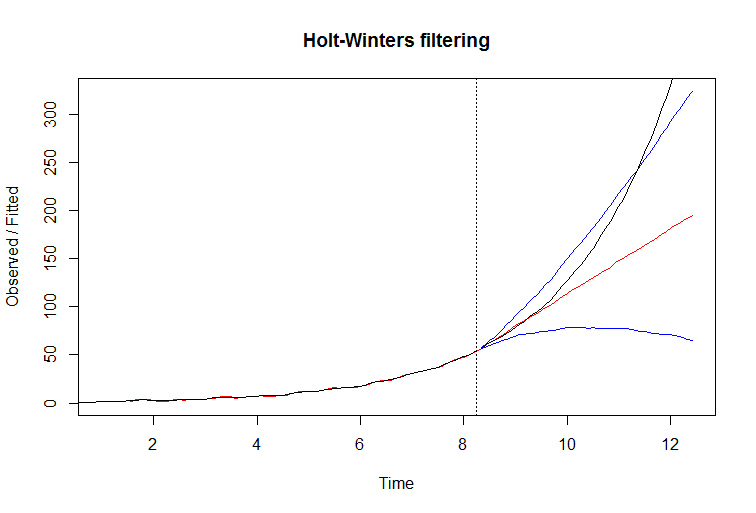
The reason why it fails is easy to see by examining Holt Winters model equations. It extracts the slope from past, and extends to future. This works very well when the slope is stable, but when it is consistently growing the filter can't keep up, it's one step behind and the effect accumulates into an increasing forecast error.
R code:
t=1:150
a = 0.04
x=ts(exp(a*t)+sin(t/5)*sin(t/2),deltat = 1/12,start=0)
xt = window(x,0,99/12)
plot(xt)
(m <- HoltWinters(xt))
plot(m)
plot(fitted(m))
xp = window(x,8.33)
p <- predict(m, 50, prediction.interval = TRUE)
plot(m, p)
lines(xp,col="black")