Tha accepted answer from @Anthony makes the main point: your results have tickled a bug in the software you used.
This is a bundle of extra comments using the sample given in Edit II as a sandbox.
As in the distribution histogram shown in the question for other data, you have a spiky, roughly U-shaped distribution. A logarithmic transformation will not help with such a distribution. It will just make it look and be a worse fit. The spikes will remain spikes. Here is a graph of the distribution.
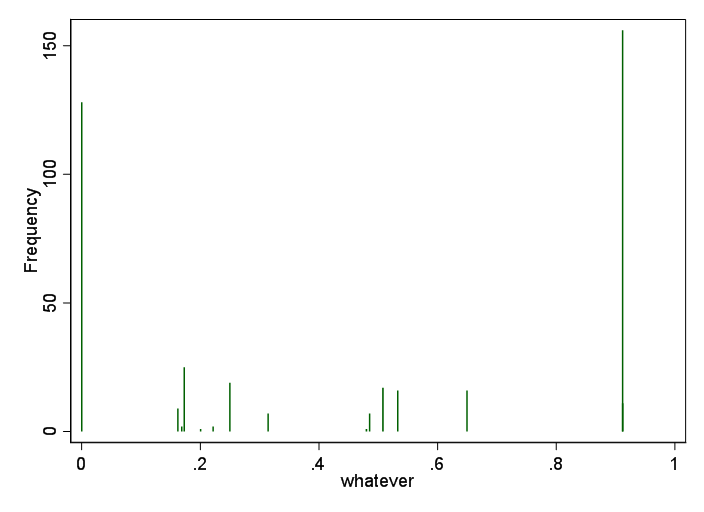
There should be a story behind the repeated values: in a sample of 417, you have only a small number of distinct values.
whatever | Freq. Percent Cum.
------------+-----------------------------------
.0001 | 128 30.70 30.70
.1622 | 9 2.16 32.85
.1687 | 2 0.48 33.33
.1729 | 25 6.00 39.33
.2005 | 1 0.24 39.57
.2216 | 2 0.48 40.05
.2498 | 19 4.56 44.60
.3143 | 7 1.68 46.28
.48 | 1 0.24 46.52
.4854 | 7 1.68 48.20
.5078 | 17 4.08 52.28
.5328 | 16 3.84 56.12
.6496 | 16 3.84 59.95
.9119 | 156 37.41 97.36
.912 | 11 2.64 100.00
------------+-----------------------------------
Total | 417 100.00
That aside, for these data I get the following results for moment-based statistics in Stata. The definitions used (on which a Gaussian/normal would have skewness 0 and kurtosis 3) are documented on p.9 of stata.com/manuals/rsummarize.pdf Other formulas exist but for this sample size they shouldn't make that much difference.
----------------------------------------------------------
n = 417 | mean SD skewness kurtosis
----------+-----------------------------------------------
whatever | 0.473 0.398 -0.027 1.243
----------------------------------------------------------
Some people like to work with so-called excess kurtosis, subtracting 3. Here that would be $−$1.757.
In terms of kurtosis the example data here are clearly non-normal. Any test based on skewness and kurtosis should therefore reject a null of normality. For context, minimum possible kurtosis is 1 (excess kurtosis $−$2); that minimum is attainable if half the data are equal to a maximum and half equal to a minimum (e.g. probability of 0 and of 1 both 0.5). Kurtosis just above 1 is expected for a U-shaped distribution, as here.