The easiest way to answer your question is to understand that roughly the data sets are often categorized as cross-sectional, time series and panel. Cross-sectional regression is a go-to tool for the cross-sectional data sets. This is what most people know and refer to with a term regression. Time series regression is sometimes applied to time-series, but time series analysis has a wide range of tools beyond the regression.
Example of cross-sectional data is $(x_1,y_1),(x_2,y_3),\dots,(x_n,y_n)$, where $x_i,y_i$ are weights and heights of randomly picked students in a school. When a sample is random we can often run a linear regression $y\sim x$ and get reliable results, to maybe predict height $\hat y$ of a student in this school knowing only student's weight $x$.
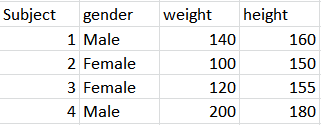
If the sample wasn't random, then the regression may not work at all. For instance, you picked only girls in first grade to estimate the model, but you have to predict the height of a male 12th grader. So, the regression has its own issues even in the cross-sectional setup.
Now, look at time series data, it could be $x_t,y_t$ such as $(x_1,y_1),(x_2,y_3),\dots,(x_n,y_n)$, where $t$ the month of a year, and $x,y$ are still weight and height but of a particular student in this school.
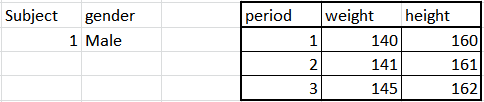
Generally, regression doesn't have to work at all. One reason is that indices $t$ are ordered. So your sample is not random, and I mentioned earlier that regression prefers a random sample to work properly. This is a serious issue. Time series data tend to be persistent, e.g. your height this month is highly correlated to your height next month. In order to deal with these issues time series analysis was developed, it included the regression technique too, but it has to be used in certain ways.
The third common dataset type is a panel, particularly, the one iwth longitudinal data. Here, you may get several snapshots of weight and height variables for a number of students. This dataset may look like waves of cross-sections or a set of time series.
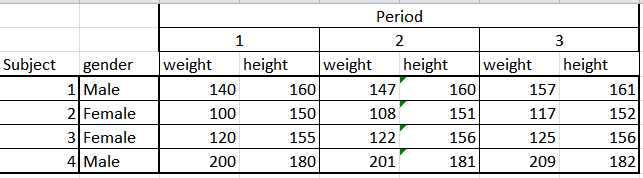
Naturally, this can be more complicated than previous two types. Here we use panel regression and other special techniques developed for panels.
Summarizing, the reason why time series regression is considered as a distinct tool compared to cross-sectional regression is that time series present unique challenges when it comes to independence assumptions of regression technique. Particularly, due to the fact that unlike in cross-sectional analysis, the order of observations matters, it usually leads to all kinds of correlation and dependence structures, which may sometimes invalidate application of regression techniques. You have to deal with dependence, and that's exactly what time series analysis is good at.
Predictability of Asset Prices
Also, you're repeating a common misconception about stock markets and asset prices in general, that they cannot be predicted. This statement is too general to be true. It's true that you can't outright predict next tick of AAPL reliably. However, it's a very narrow problem. If you cast your net wider you'll discover a lot of opportunities to make money using for all kinds of forecasting (and time series analysis in particular). Statistical arbitrage is one such field.
Now, the reason why asset prices are hard to predict in near term is due to the fact that a large component of price changes is new information. The truly new information that cannot be realistically devised from the past is by definition impossible to predict. However, this is an idealized model, and a lot of people would argue that the anomalies exist that allow for persistence of state. This means that the part of price change can be explained by the past. In such cases time series analysis is quite appropriate because it precisely deals with persistence. It separate new from old, new is impossible to predict, but old is dragged from the past into the future. If you can explain even a little bit, in finance it means that you may be able to make money. As long as the price of the strategy built on such forecasting covers the income generated by it.
Finally, take a look at the economics nobel prize in 2013: "it is quite possible to foresee the broad course of these prices over longer periods, such as the next three to five years." Take a look at Shiller's nobel lecture, he discusses forecastability of asset prices.