Consider the following dataset:
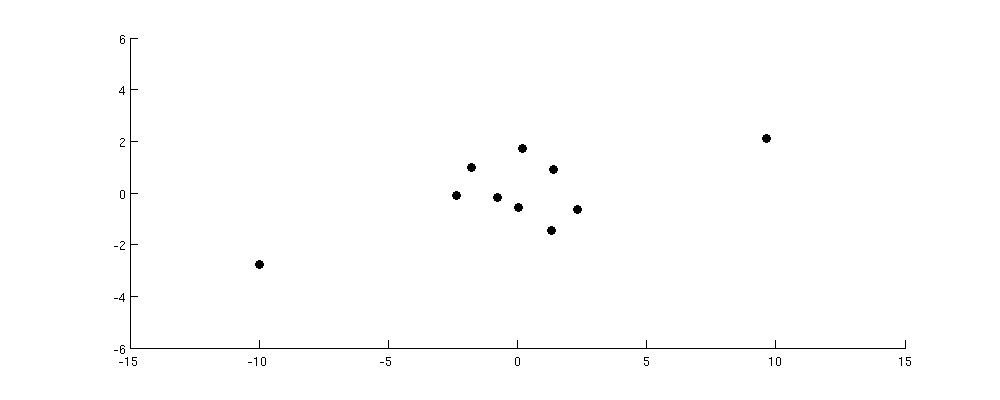
PC1 axis is maximizing the variance of the projection. So in this case it will obviously go diagonally from lower-left to upper-right corner:
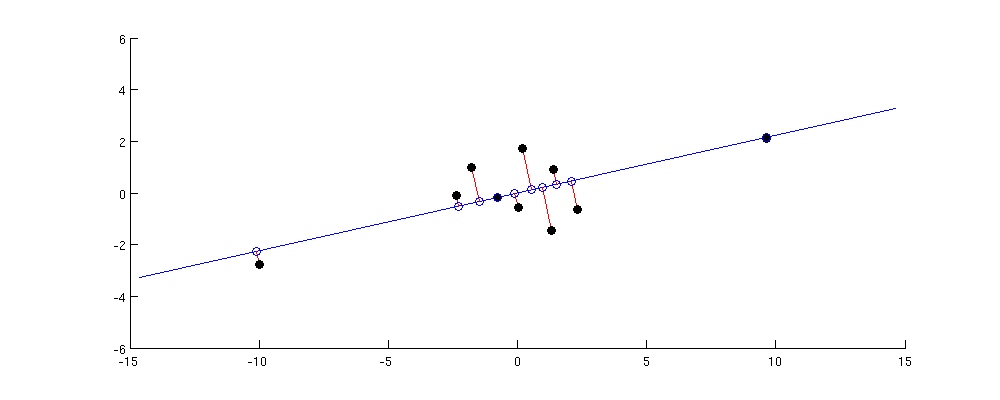
The largest pairwise distance in the original dataset is between these two outlying points; notice that it is almost exactly preserved in the PC1. Smaller but still substantial pairwise distances are between each of the outlying points and all other points; those are preserved reasonably well too. But if you look at the even smaller pairwise distances between the points in the central cluster, then you will see that some of them are strongly distorted.
I think this gives the right intuition: PCA finds low-dimensional subspace with maximal variance. Maximal variance means that the subspace will tend to be aligned such as to go close to the points lying far away from the center; therefore the largest pairwise distances will tend to be preserved well and the smaller ones less so.
However, note that this cannot be turned into a formal argument because in fact it is not necessarily true. Take a look at my answer in What's the difference between principal component analysis and multidimensional scaling? If you take the $10$ points from the figures above, construct a $10\times 10$ matrix of pairwise distances and ask what is the 1D projection that preserves the distances as close as possible, then the answer is given by MDS solution and is not given by PC1. However, if you consider a $10\times 10$ matrix of pairwise centered scalar products, then it is in fact best preserved precisely by PC1 (see my answer there for the proof). And one can argue that large pairwise distances usually mean large scalar products too; in fact, one of the MDS algorithms (classical/Torgerson MDS) is willing to explicitly make this assumption.
So to summarize:
- PCA aims at preserving the matrix of pairwise scalar products, in the sense that the sum of squared differences between the original and reconstructed scalar products should be minimal.
- This means that it will rather preserve the scalar products with largest absolute value and will care less about those with small absolute value, as they add less towards the sum of squared errors.
- Hence, PCA preserves larger scalar products better than the smaller ones.
- Pairwise distances will be preserved only as much as they are similar to the scalar products which is often but not always the case. If it is the case, then larger pairwise distances will also be preserved better than the smaller ones.