TL;DR: you can't run seasonal models with a seasonality of 365 days in ETS
. Use stlf
instead.
The best approach would be to specify your original time series (in your case, precipitacaoTotal
) as seasonal. This is what you could do for monthly seasonality:
library(fable)
set.seed(1)
foo <- as_tsibble(ts(rnorm(1000),frequency=12))
foo %>% model(ETS(value~season(method="A"))) %>% forecast(h="3 years") %>% autoplot(foo)
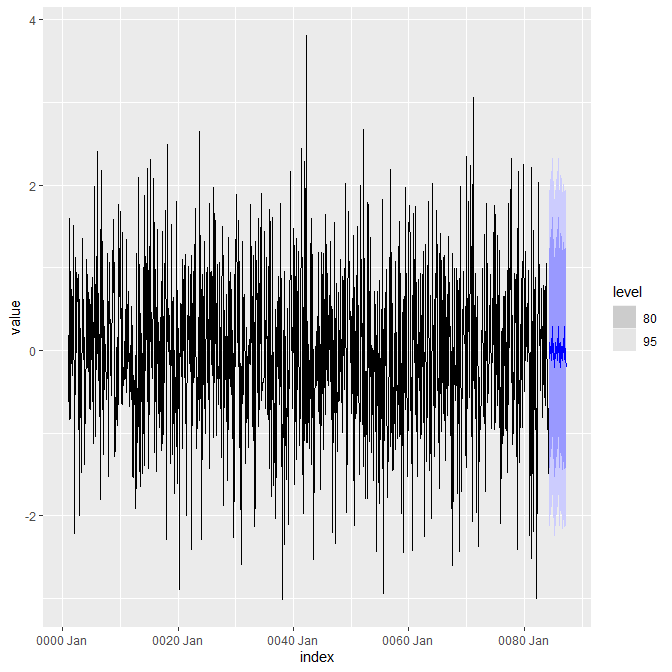
Since I forced seasonality using season(method="A")
, we get a (pedagogically useful, but of course nonsensical) seasonal forecast. If we let ETS
decide on a model on white noise, it would of course not choose a seasonal one.
However, this will not work for longer periods. If we use the same idea with frequency=365
,
set.seed(1)
as_tsibble(ts(rnorm(1000),frequency=365)) %>% model(ETS(value))
we get a rather unhelpful error message (formatted):
# A mable: 1 x 1
`ETS(value)`
<model>
1 <NULL model>
Warning message:
1 error encountered for ETS(value)
[1] .data contains implicit gaps in time.
You should check your data and convert implicit gaps into explicit
missing values using `tsibble::fill_gaps()` if required.
I don't quite see where ETS
sees implicit gaps, and have pinged the maintainers of fable
.
However, the underlying reason is probably that ETS
does not support seasonal periods longer than 24 periods: running
set.seed(1)
as_tsibble(ts(rnorm(1000))) %>% model(ETS(value~season(period=365)))
yields the much more informative warning message
# A mable: 1 x 1
`ETS(value ~ season(period = 365))`
<model>
1 <ETS(A,N,N)>
Warning message:
Seasonal periods (`period`) of length greather than 24 are not supported by ETS.
Seasonality will be ignored.
This is actually the same behavior as in the older forecast
package. Running
library(forecast)
set.seed(1)
ets(ts(rnorm(1000),frequency=365))
yielded (among other outputs):
I can't handle data with frequency greater than 24.
Seasonality will be ignored. Try stlf() if you need seasonal forecasts.
And this actually makes a lot of sense. In exponential smoothing (whether in a state space framework or otherwise), having a seasonality of $k$ periods means you need to estimate $k$ initial conditions. 365 initial conditions is a lot. You would overfit massively. So go with stlf
instead.
precipitacaoTotal
time series has a frequency of 365. Would you be open to a solution in base R and theforecast
package? I do not use tidyverse tools, I find them a completely useless piece of extra complexity I would need to wade through. In any case, do you have a specific reason to specify the error/trend/seasonality structure, rather than letETS
decide? It's very good at that. $\endgroup$