Here is an example that can be pasted to an IPython prompt and generate an image like below (it uses random data):
import numpy as np
import matplotlib
import matplotlib.pyplot as plt
#Make a random array and then make it positive-definite
num_vars = 6
num_obs = 9
A = np.random.randn(num_obs, num_vars)
A = np.asmatrix(A.T) * np.asmatrix(A)
U, S, V = np.linalg.svd(A)
eigvals = S**2 / np.sum(S**2) # NOTE (@amoeba): These are not PCA eigenvalues.
# This question is about SVD.
fig = plt.figure(figsize=(8,5))
sing_vals = np.arange(num_vars) + 1
plt.plot(sing_vals, eigvals, 'ro-', linewidth=2)
plt.title('Scree Plot')
plt.xlabel('Principal Component')
plt.ylabel('Eigenvalue')
#I don't like the default legend so I typically make mine like below, e.g.
#with smaller fonts and a bit transparent so I do not cover up data, and make
#it moveable by the viewer in case upper-right is a bad place for it
leg = plt.legend(['Eigenvalues from SVD'], loc='best', borderpad=0.3,
shadow=False, prop=matplotlib.font_manager.FontProperties(size='small'),
markerscale=0.4)
leg.get_frame().set_alpha(0.4)
leg.draggable(state=True)
plt.show()
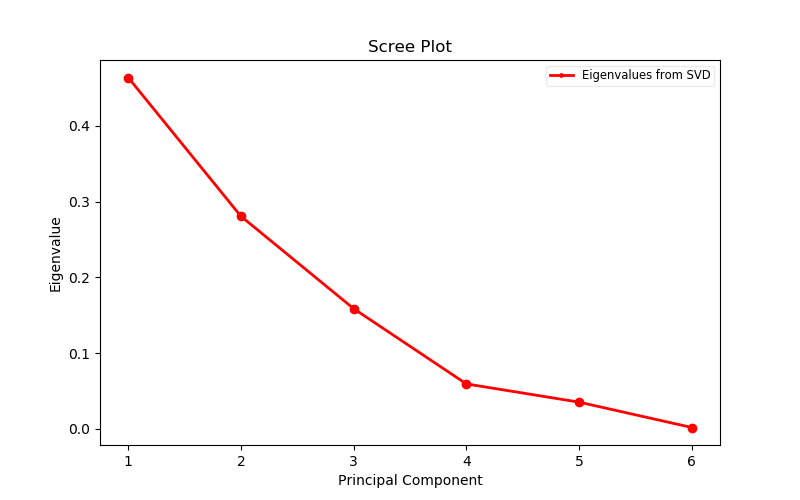
S
, if it is not already a diagonal, square it, sort it in decreasing order, take the cumulative sum, divide by the last value, then plot it. $\endgroup$[U,S,V] = svd(X);S = cumsum(sort(diag(S).^2,1,'descend'));S = S ./ S(end);plot(S);
$\endgroup$