TL;DR No, it is not correct and few things can be improved.
Poor performances is due to bugs in the code: using CDF rather then PDF and drawing new values independently of previous ones. Your implementation would return wrong results.
while i < n:
new_x = random()
Do you know what algorithm Python uses for random generation in random()
function? In many cases you should not use the default pseudo-random generators for statistical purposes (e.g. this comment about C++ rand()
). Hopefully Python uses Mersenne Twister algorithm that is pretty good and widely used, but this is worth checking in advance.
Moreover, what you implemented is closer to random-walk Metropolis algorithm (check e.g. Monte Carlo Statistical Methods book by Robert and Casella), where new value is drawn independent of previous draw, rather then dependent as in the Metropolis algorithm. This is also the reason for poor performance. You should rather be using something like
new_x = current_x + random.uniform(-eps, eps)
where eps
is some small constant. Random-walk Metropolis algorithm has higher rejection rate then the Metropolis algorithm. There is also a problem in next line:
new_f = CDF(new_x)
current_f = CDF(current_x)
This should be probability density functions, or probability mass functions, not cumulative distribution functions. Check What is the equivalent for cdfs of MCMC for pdfs? for learning more about MCMC-like algorithms for CDF's.
Notice that when using Metropolis algorithm with CDF you receive sample that is strongly biased against higher values since $\Pr(X \le x)$ will be always higher for larger $x$'s (with probability equal to $1$ for $\infty$). If you used PDF you would be drawing values with associated higher probability more often then those with associated lower probabilities (so $-\infty$ and $\infty$ would be both equally unlikely). This is illustrated on the plots below where Metropolis algorithm is used to draw from standard normal distribution using PDF (left), or with CDF (right). As you can see on the plots, when using CDF it is going upwards and accepting even very unlikely values of $X \ge 4$.
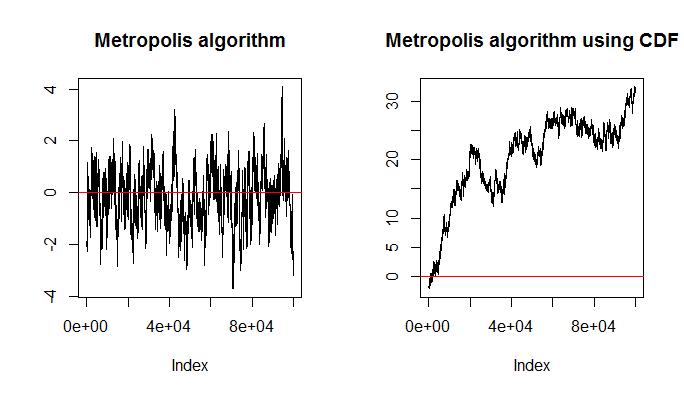
Besides of that, you could improve few things.
a = abs(new_f / current_f)
Why are you using abs()
in here? You are dividing something that is positive, by something that is also positive, so the result cannot be negative. There is no need for taking absolute value in here.
transition_prob = min(1, a)
By definition of Metropolis algorithm there is min()
in here but notice that in the next step you are comparing a
to $\mathcal{U}(0,1)$ random variable that cannot be greater then $1$, so min()
in the previous step does not change anything about values of a
greater than $1$ since they will be accepted despite of that. Check this entry from Darren Wilkinson's blog for learning more about examples of implementing Metropolis algorithm.
The rest is fine.
- In the sampling I have rejected 49% of actual function evaluations. Those rejected evaluations could have provided me a bigger insight of
the objective function. Is that so?
You reject those values because in the end you want to have sample where each value appears with similar probability as in your target distribution. If you accepted everything, then the values would not appear with correct probabilities unless you are sampling from the target distribution.
- Is MCMC better than MC for "mapping" a very complicated black box function, given that I would reject many costly function evaluations?
What do you mean by MC? Monte Carlo? There is a number of Monte Carlo algorithms, with MCMC algorithms belonging to this group. Yes, there are Monte Carlo algorithms that are more efficient then Metropolis algorithm, but I'm not sure what you mean in here. Also, if you can sample directly from your target distribution than obviously this is more efficient then using Metropolis algorithm - if this is what you meant.
EDIT
Is seems that in your code function CDF()
is in fact an inverse of cumulative distribution function (a.k.a. quantile function). In this case there is no reason to use Metropolis algorithm at all since you can simply use inverse transform sampling to generate samples from your distribution directly. What you need to do is to take uniformly distributed random variable $U$ and pass it through the inverse CDF. This is computationally efficient, one of the most simple and basic ways of generating draws from non-uniform random variables. We do not use quantile function in Metropolis algorithm the same as we do not use CDF. If you used quantile function in Metropolis algorithm, then what would you be telling to the algorithm is "give me larger values of $X$ with higher probability", rather then drawing more probable values with higher probability.