Here is an explanation of the mathematics behind a case-cohort study.
Below we see a sketch for a simulation of data in a cohort study among 2500 people with an 'outcome' sick vs not sick and this is being studied as a function of a variable 'type' which has 3 levels.
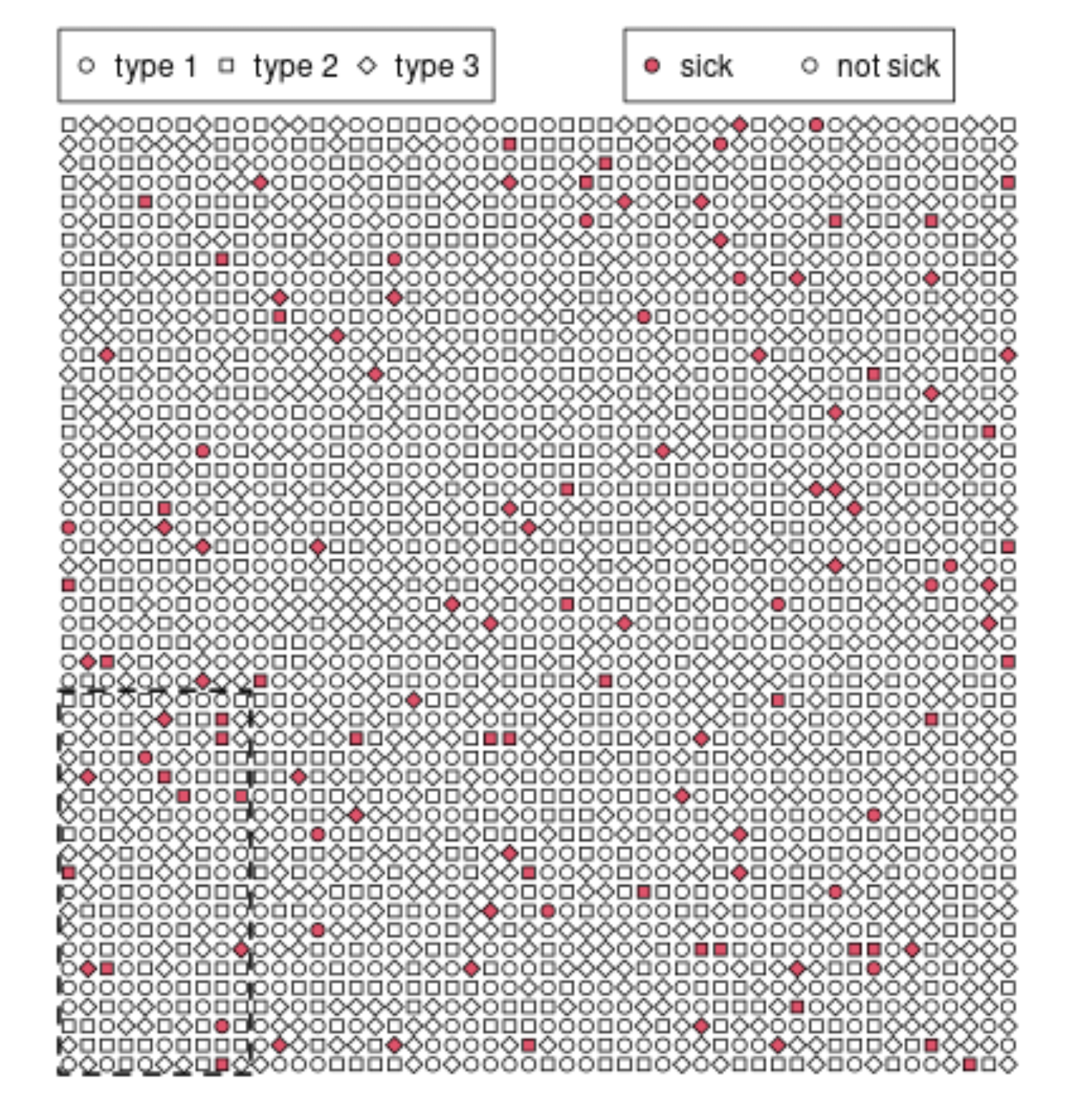
If we study the entire cohort then we would have a contingency table like
$$\begin{array}{r|ccc}
&\text{type 1}&\text{ type 2}&\text{ type 3} \\ \hline
\text{sick}&19&43&54 \\
\text{not sick}&840&798&746\\
\\
\text{sum}&859&841&800\\
\end{array}$$
This requires to measure the types among all 2500 patients.
The alternative with a case-cohort study is to have a sub-cohort like the 200 people in the 10 by 20 square in the lower left corner and only determine the types in the sub cohort and the positive cases. Then the table will look like:
$$\begin{array}{r|ccc|ccc}
&\rlap{\text{rest of cohort}}&&&\rlap{\text{sub cohort}}\\
&\text{type 1}&\text{ type 2}&\text{ type 3}
&\text{type 1}&\text{ type 2}&\text{ type 3} \\ \hline
\text{sick}&17&35&50 &2&8&4\\
\text{not sick}&\color{pink}{768}&\color{pink}{732}&\color{pink}{698}&72&66&48\\
\\
\text{sum}&\color{pink}{785}&\color{pink}{767}&\color{pink}{748}&74&74&52\\
\end{array}$$
Here, the pink numbers are not observed. And we only know the 12 data values in black.
These values can be modelled based on the probabilities of the disease occuring or not for the types, $p_1,p_2,p_3$, as well as the odds of being outside the sub-cohort $o_{\neg sub}$ and the numbers in the subcohort $n_1,n_2,n_3$.
$$\begin{array}{r|ccc|ccc}
&\rlap{\text{rest of cohort}}&&&\rlap{\text{sub cohort}}\\
&\text{type 1}&\text{ type 2}&\text{ type 3}
&\text{type 1}&\text{ type 2}&\text{ type 3} \\ \hline
\text{sick}&p_1n_1o_{\neg sub}&p_2n_2o_{\neg sub}&p_3n_3o_{\neg sub} &p_1n_1&p_2n_2&p_3n_3 \\
\text{not sick}&-&-&-&(1-p_1)n_1&(1-p_2)n_2&(1-p_3)n_3 \\
\hline
\text{total}&-&-&-&n_1&n_2&n_3
\end{array}$$
The above is the case for a contingency table, the same may be done for a proportional hazards model. Now the computations are potentially not including those bins anymore (e.g. if the variables are continuous instead of categorical), but then we have all the $n_i=1$ and it works the same. For a relative hazards model, you compute the odds of occuring in a specific individual given that somebody got sick, and you see that there is an additional factor $o_{\neg sub}$ if that individual is outside the sub-cohort.
(A)
can I weight the sample based on prevalence or incidence rates from external sources?
The weighing occurs based on the odds of being in the sub-cohort.
(B)
Can this study be considered a case-cohort study?
The case-cohort study selects a sub-cohort that is independent from being sick or not. Your controls may have been selected depending on whether they are not sick. Possibly you may perform some similar corrections but it is not the same. You can not make the 2nd table like above where you can compute the prevalences of the types, and you can not make the step from contingency to proportional hazards in case of continuous variables (where there are no categories and no clearly defined prevalences).
(C)
is there an analogous approach in survival analysis
Because it is simpler, I have made an example with a contingency table. So that is one analogous approach. The problem with this alternative is that it doesn't work with continuous variables and with variable exposure times among subjects.
Beyond that, there are many others. Any alternative to relative hazards (e.g. an accelerated time model) can give an analogues approach. You just add the factor $o_{\neg sub}$ for the risk.
Code for image
set.seed(1)
n = 50
type = sample(1:3, n^2, replace = TRUE)
x = rep(1:n,n)
y = rep(1:n,each=n)
p = type/50
sick = rbinom(n^2,1,p)
par(mar = c(1,1,1,1))
plot(x,y,pch=20+type, cex = 1,
xlab = "", ylab = "",
xaxt = "n", yaxt = "n",
bg = 0+2*sick, bty = "n", xlim = c(0,n+5), ylim = c(0,n+5))
legend(0.5,n+5, c("type 1", "type 2", "type 3"), pch = c(21,22,23), ncol = 3)
legend(30,n+5, c("sick", "not sick"), pt.bg = c(2,0), pch = 21, ncol = 2)
lines(c(0,10,10,0,0)+0.5,
c(20,20,0,0,20)+0.5, lty = 2, lwd = 2)
sub = (x<=10)*(y<=20)
table(sick, paste0(sub,type))
table(sick, paste0(type))
time = 0
) for the survival analysis? Date of birth, date of entry into study, some calendar date, ...? What/how many predictors are you intending to use? How many events (development of disease) are there? That's much more important than the number of cases. $\endgroup$