Suppose I take Success to mean Female. Then the number of Females
in a random sample from University A is $X \sim \mathsf{Binom}(n=100,p=0.7)$
and the number of Females in a random sample from University B is
$Y \sim \mathsf{Binom}(n=100,p=0.3).$
Try one test. Let's try using prop.test
in R to analyze one such experiment with 200 students:
set.seed(2020)
x = rbinom(1, 100, .7); y = rbinom(1, 100, .3)
x; y
[1] 68
[1] 32
prop.test(c(x,y),c(100,100), cor=F)
2-sample test for equality of proportions
without continuity correction
data: c(x, y) out of c(100, 100)
X-squared = 25.92, df = 1, p-value = 3.559e-07
alternative hypothesis: two.sided
95 percent confidence interval:
0.2307018 0.4892982
sample estimates:
prop 1 prop 2
0.68 0.32
So in this particular experiment, the test finds very strong evidence
to reject $H_0: p_a = p_b$ with P-value very near $0.$ [Use of a continuity
correction is not useful for samples of size 100, so I used parameter
cor=F
in prop.test
to disallow continuity correction.]
Then the question is whether I somehow got an outrageously atypical
pair of samples in the example above, or whether prop.test
really
does have good power to detect the large difference in the proportions
of Female students at the two universities, based on samples of $n_a = n_b = 100$ from each university.
Simulate 100,000 tests to estimate power. By doing the experiment 100,000 times, I can closely estimate the
power of this test. [Computations in R.]
set.seed(722)
pv = replicate(10^5, prop.test(c(rbinom(1,100,.3),
rbinom(1,100,.7)), c(100,100),cor=F)$p.val)
mean(pv <= .05)
[1] 0.99996
The answer is that the power of the test to detect the difference in
proportions (at the 5% level) is above 99%. So it would be extremely rare
for such an experiment not to show a difference in proportions.
Specifically, the answer is 'a probability of almost 1'.
There are several versions of this test (depending on whether a normal
approximation is involved, whether a continuity correction is used,
and whether the test uses a 'pooled' standard error (under the null
hypothesis that proportions are equal). Not knowing the version of
the test you will use, I can't give an algebraic solution. (Also, this
is a 'self-study' problem and you have not shown what you have tried,
so I have no way to guess what approach you might be planning/expected to use.)
Lower bound on power. Here is one possible approach that does not use simulation:
If we have $X=60, Y=40,$ then prop.test
rejects, so it will
also reject for more extreme differences such as $X=61, Y=39,$
and so on. [You might use your favorite test here instead of R's implementation of prop.test
.]
prop.test(c(40,60), c(100,100), cor=F)$p.val
[1] 0.004677735
However the exact binomial probability of $P(X \ge 60, Y \le 40)
= P(X \ge 50)P(Y \le 40) = 0.9875.$ So that gives a pretty good idea
that rejection is nearly certain.
pbinom(40, 100, .3)*(1-pbinom(40, 100, .7))
[1] 0.9875016
The plot below shows that PDFs of $\mathsf{Binom}(100, 0.3)$ and $\mathsf{Binom}(100, 0.7)$ hardly overlap.
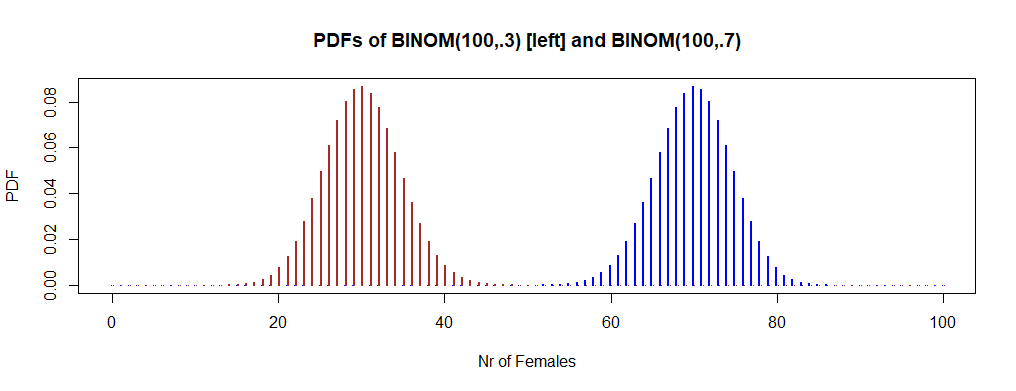
x = 0:100; pdf.x = dbinom(x, 100, .7)
y = 0:100; pdf.y = dbinom(y, 100, .3)
hdr="PDFs of BINOM(100,.3) [left] and BINOM(100,.7)"
plot(x-.1, pdf.x, type="h", col="blue", lwd=2,
ylab="PDF", xlab="Nr of Females", main=hdr)
points(y+.1, pdf.y, type="h", col="brown", lwd=2)
Addendum, per Comment: The answer is about 12% power.
set.seed(723)
pv = replicate(10^5, prop.test(c(rbinom(1,100,.48),
rbinom(1,100,.53)), c(100,100),cor=F)$p.val)
mean(pv <= .05)
[1] 0.11845