In classical estimation theory, i.e. estimation of non random parameter, an efficient estimator is one that is unbiased and achieves the CRLB for the parameter estimated.
CRLB gives a lower bound on the variance of the estimator i.e. no estimator in the classical estimation setting can ever have a variance less than the CRLB. If luckily we can come up with an estimator having the variance equal to the CRLB, it is called an efficient estimator.
Now even in situations where we can't meet the CRLB bound we can still come up with an estimator that has uniformly (at all points) less or equal variance as all known unbiased estimators. Such an estimator is called UMVUE.
In figure (a), $ \hat\theta_1, \hat\theta_2, \hat\theta_3 $ are all unbiased and $\hat\theta_1$ attains the CRLB. As such it is MVU as well as efficient.
In figure (b), $ \hat\theta_1, \hat\theta_2, \hat\theta_3 $ are all unbiased but none attains the CRLB. However $\hat\theta_1$ attains a lower variance than the other two. As such it is MVU but not efficient.
Therefore, efficiency implies minimum variance but not the other way round.
NOTE:
- CRLB stands for Cramer Rao Lower Bound.
- Computing the CRLB is possible only if we know the pdf of the data and the pdf follows the regularity conditions i.e. we can interchange the order of integration and differentiation. So this method is not applicable always
- UMVUE may exist irrespective of whether a CRLB is obtainable or not. For UMVUE, all that we need to see is that firstly the estimator is unbiased and secondly that it obtains a variance less than or equal to all known unbiased estimators.
- CRLB is just one of the different strategies for obtaining an MVU estimator. Other strategies include the concept of sufficient statistics such as RBLS (Rao Blackwell Lehmann Scheffe Theorem)
Refer: Estimation theory by Steven Kay 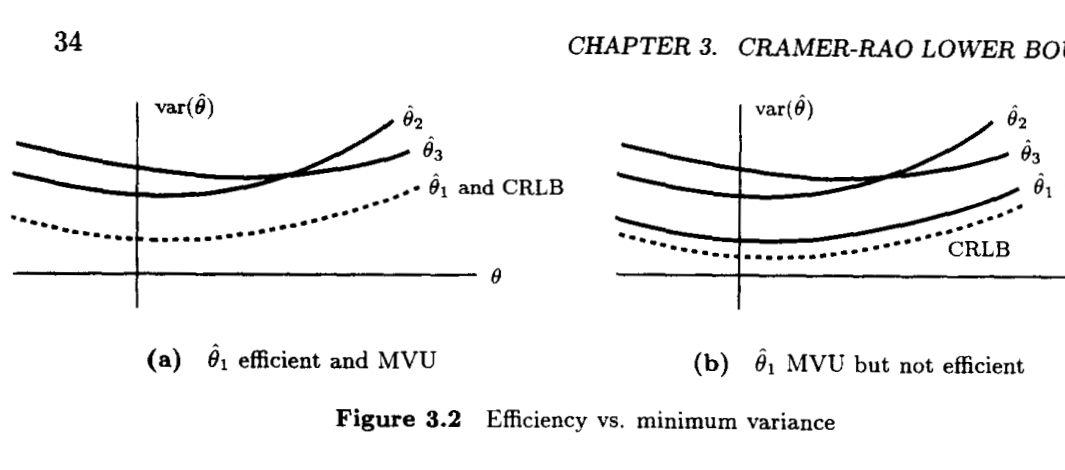