After working on the problem for a few days I finally found a paper that had an explanation that I could (somewhat) understand. The paper in question is
Approximation and Simulation of the Distribution of Scan Statistics for Poisson Processes in Higher Dimensions, Sven Erick Alm 1997
It turns out that finding an exact solution to my problem was not feasible but the paper presented an equation for calculating an approximation. An abbreviated explanation of the approximation follows.
We are scanning over a Poisson process (with the scanning process $Y(t)$) of length $T$ with a time window of size $\delta$. The maximum value found when scanning is $N$ and this can be expressed as.
$$
N = \max_{\delta\leq t\leq T}Y(t)
$$
The cumulative distribution function of $N$ can be approximated using the following expression.
$$
P(N\leq n) = P(n, \lambda\delta)e^{-(1-\frac{\lambda \delta}{n + 1})\lambda(T - \delta)p(n, \lambda\delta)}
$$
where $\lambda$ is the Poisson process intensity, $P$ is the cumulative probability function of the Poisson distribution and $p$ is the probability function of the Poisson distribution as follows.
$$
P(k, \mu) = e^{-\mu}\sum_{i=0}^{k}\frac{\mu^i}{i!}
$$
$$
p(k, \mu) = \frac{e^{-\mu}\mu^k}{k!}
$$
I compared the approximation to simulated values of $N$ with $\lambda=4$, $\delta=1$ and $T=600$. The approximation fails pretty badly for small values of $n$ (e.g. $n<5$) but appears to work fine for larger values.
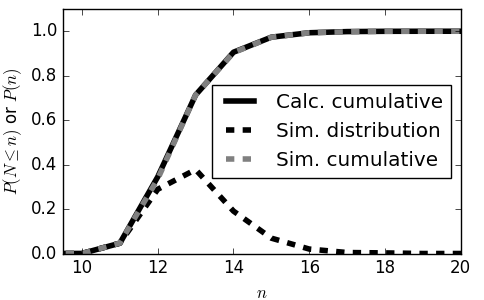
The simulation and approximation was implemented in Python and I have provided the implementation of the approximation in case it is of use to anyone.
from numpy import *
from scipy.misc import factorial
def P_mu(k, lam):
k_arr = arange(0, k + 1)
return exp(-lam) * ((lam**k_arr)/factorial(k_arr)).sum()
def p_mu(k, lam):
return (exp(-lam) * lam**k)/factorial(k)
def P_N(n, lam, int_time, total_time):
sup_part = (1.0 - (lam * int_time) / (n + 1)) * lam * (total_time - int_time) * p_mu(n, lam * int_time)
return P_mu(n, lam * int_time) * exp(-sup_part)