The article did not cluster on the DTM, but on the distance matrix as returned by dist.
The function dist
computes the euclidean distance between vectors, and kmeans
uses this measure to cluster on. Think of each document as an observation and each unique term you have as a dimension. kmeans
is comparing the distance between the high-dimensional vectors that represent the columns of the DTM and clustering vectors that are similar with one another based on the distance metric.
To visualize what dist
does, imagine a 3 dimensional box. dist
could be used to measure the straight-line distance from, say, the front, bottom, left corner to the back, top, right corner (see image).
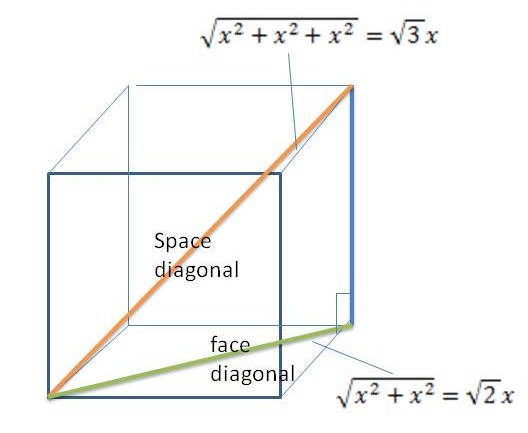
Even if the box is a perfect cube, this distance is not equal to the length of one of the sides, the same way that the diagonal of a square (green line of the bottom) is not the same length as the sides of the square. Now imagine going up into 4, 5, ... , N dimensions. The euclidean distance formula (given in the image for 2 and 3 dimensions) can be extended to give the distance between two vectors in any number of dimensions.
For an example using words, think of "the quick brown fox" as a vector with values of 1 for "the", "quick", "brown", and fox". "The lazy dog" is another vector with values of 1 for "the", "lazy", and "dog", but 0's for "quick", "brown" and "fox".
the quick brown fox lazy dog
words.1 1 1 1 1 0 0
words.2 1 0 0 0 1 1
dist(words)
words.1
words.2 2.236068
dist
gives the length of the line that would connect the vectors words.1
and words.2
in this 6 dimensional space. If we had multiple vectors in this space, dist
would return a matrix (technically my example returned a 1 x 1 matrix) of the distance between each vector and every other vector in the space, similar to what a covariance matrix does. Vectors that are more similar can be thought of as "closer together" than vectors that are different. We can go back to the cube example, but still with words, now:
front back top bottom left right
V1 1 0 0 1 1 0
V2 0 1 1 0 0 1
V3 1 0 0 1 0 1
Just like in the picture above, the front, bottom, left vector (V1) is as far away (or as different) as possible than the back, top, right vector (V2). We expect that V1 and V2 will be the furthest from each other, and since V1 and V3 have 2 words ("front" and "bottom") in common, we expect the V1 to V3 distance to be less.
dist(a)
V1 V2
V2 2.449490
V3 1.414214 2.000000
We were correct. The V1 to V2 distance is 2.44, which is greater than the V1 to V3 distance (1.141) and V2 distance to V3 distance (2).
This matrix of distances is what kmeans
is using to cluster.