This is merely the standard logistic distribution.
We can derive this in a few lines. Let $U\sim \text{Unif}(0,1)$
Let $X = 1/U - 1$.
$F_X(x)=P(X\leq x) = P(1/U-1 \leq x) = P(U\geq 1/(x+1)) = 1-1/(x+1)\,,x>0$
Hence $f_X(x) = 1/(x+1)^2\,,\: x>0\,.$ (This is a shifted Pareto(1))
Now let $Y=\log(X)$. Then
$P(Y\leq y) = P(\log(X)\leq y) = P(X \leq \exp(y)) = 1-\frac{1}{1+\exp(y)} = \frac{\exp(y)}{1+\exp(y)}$
which is the cdf of a standard logistic distribution.
(Such a method of generating values from the logistic distribution corresponds to the inverse-cdf method.)
It is symmetric but has exponential tails. It is fairly similar to a normal in the between the quartiles - or perhaps out a bit further, say the middle 75% or so - but not at all close to the normal distribution in the tails:
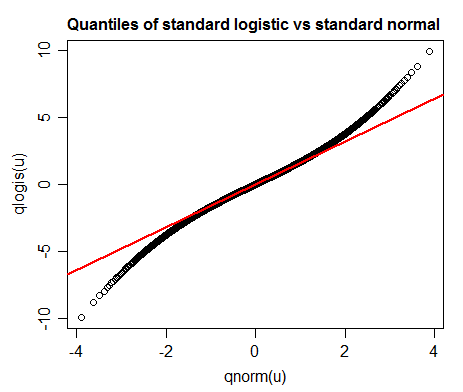
(the line has slope 1.6, approximately the ratio of the interquartile ranges)
The statement that averages converge* to a normal is hardly news; you don't need to be at all close to a normal to have that; the CLT applies quite widely.
* however the statement as given is strictly incorrect. Standardized averages converge to a standard normal by the CLT. Raw averages actually converge to a spike at 0.