I would strongly recommend that you pick up a copy of Little (2013)--there's simply too much detail to describe about how I would revise your model to accomplish your empirical goals, but Little would be a good (and comprehensive, yet accessible place to start). Here, though, are some broad ideas of how your model development strategy could proceed:
Specifying Repeated Measure Error Structure
In order to capture the repeated-measures aspect of your design, you will need to model all 8 "replicates" of Understanding, Dread, and Unknown (i.e., 8 Understanding factors, 8 Dread factors, 8 Unknown Factors). The trick to modelling the dependency in each assessment of each factor is to correlate the error terms of the same item across repeated measurements. I've included a diagram of a simplified example, of how you would do this with 3 "replicates" of one of your factors--you'll need to do this for all of your factors, and across all "replicates" of each factor. For sake of clarity, I've color-coded related residual covariances (i.e., for the same item across replicates).
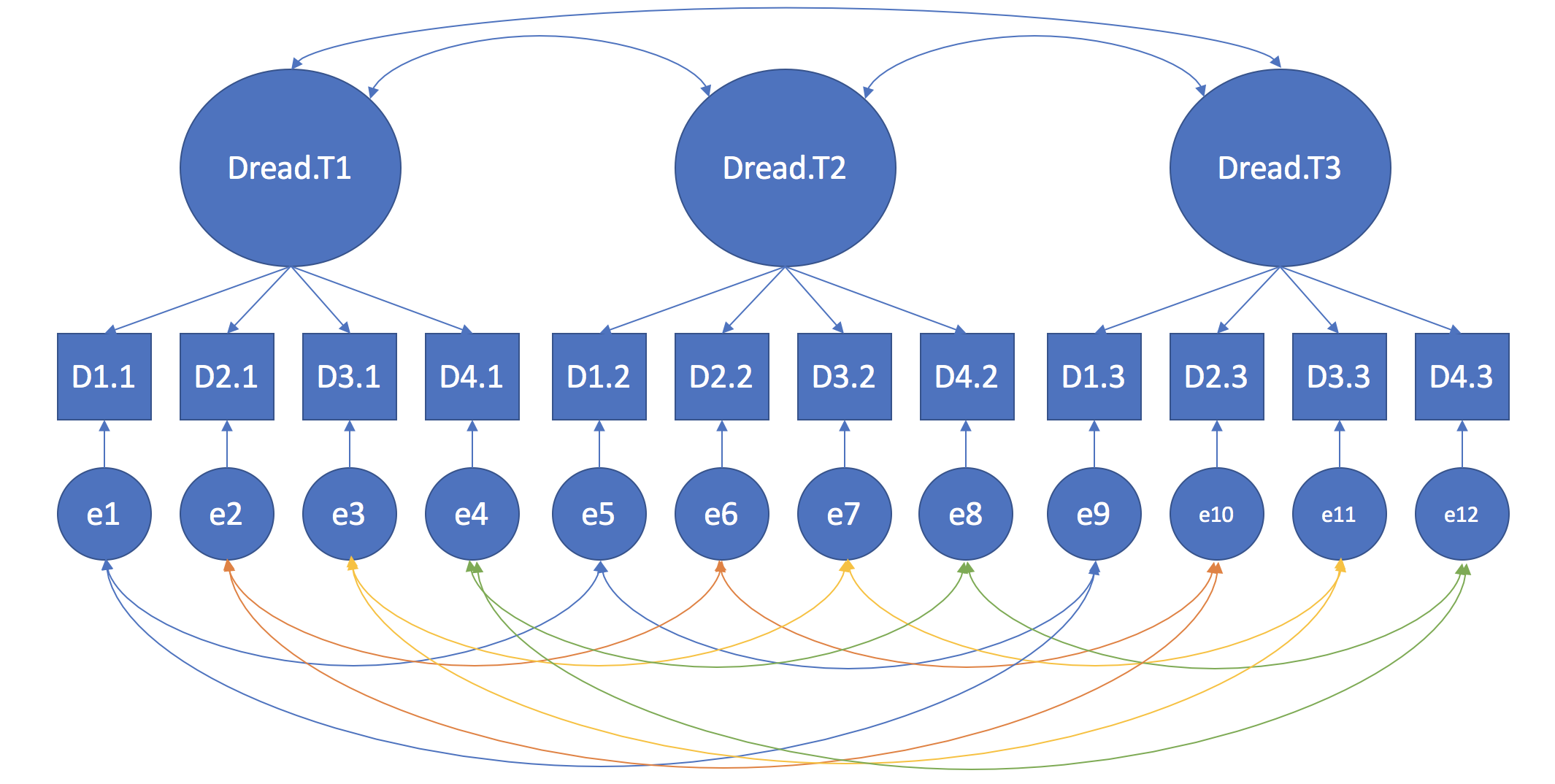
Establish Group and Longitudinal Invariance
Using an observed covariate to capture group differences (i.e., for structure.0, an approach called MIMIC modelling) makes a lot of assumptions about the equivalence of measurement (i.e., measurement invariance) between the groups. For better or worse, people make these sorts of assumptions all the time when they run a generic t-test or ANOVA. But assuming measurement invariance--rather than evaluating it--when using SEM defeats the purpose of using a latent variable approach (in my opinion). You want to examine whether your manipulation affected levels of your mediator/outcome variables, but those inferences are only valid if your manipulation did not alter the measurement makeup of those latent factors. Vandenberg and Lance (2000) have a nice review paper on measurement invariance, and Little (2013) discusses how to go about this as well.
Things get more complicated, however, with your longitudinal data; you must also ensure that the measurement of your latent factors is invariant across time points, in addition to being invariant across each group. Only then can you be sure that your manipulation is affecting latent mean levels--and not the measurement--of your factors.
Model Simplification
Assuming your group and longitudinal invariance checks pan out, you can then go about testing whether latent means vary across your manipulated groups and/or replicates. In order to simply your models a bit (multi-group models for all of these replicates will be asking a lot of your data), you might consider (1) testing whether simplex structure (see Little, 2013) is a viable parsimonious representation of the associations within a factor over time, and (2) testing whether effects of your manipulation are consistent across a factor over time. You can then go about testing whether latent means for a given factor are reasonably equal between groups in the normal SEM fashion, first by freely estimating those means between groups, then constraining them to equality, and performing a nested model comparison between the two.
References
Little, T. D. (2013). Longitudinal structural equation modeling. New York, NY: Guilford Press.
Vandenberg, R. J., & Lance, C. E. (2000). A review and synthesis of the measurement invariance literature: Suggestions, practices, and recommendations for organizational research. Organizational Research Methods, 3, 4-70.