If you are working on time series data or 1D univariate sequence data, a possible package available in R, Python, and Matlab is Rbeast
(https://github.com/zhaokg/Rbeast). It is a Bayesian changepoint detection algorithm that can handle missing data; it can also decompose time series into periodic and trend components, if needed. Below are some quick examples using the Nile river flow and COVID-19 daily new cases data:
library(Rbeast)
#### The full time series ####
plot( beast(Nile) , main='Changepoint analysis for the full dataset')
####Introduce NAs at 40 random locations out of 100####
set.seed(1234)
NileNA = Nile
NileNA[ sample(length(NileNA),40) ]= NA
plot(beast(NileNA), main='Changepoint analaysis with 40 missing datapoints')
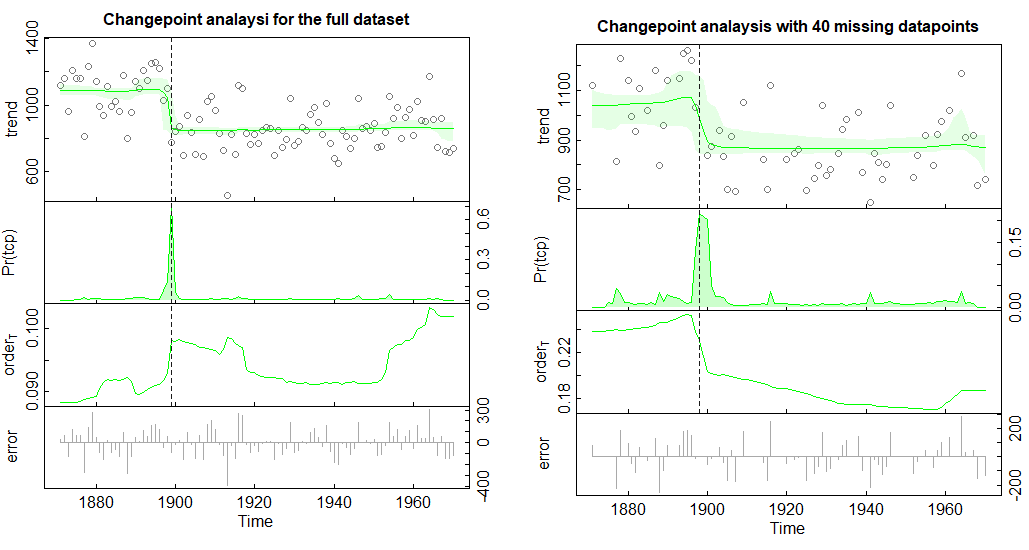
beast
estimates the probability of changepoint occurrence over time, which is the green Pr(tcp) curve above. The peak corresponds to the region/point where the changepoints are most likely to occur.
###covid19 is a daily time series with a weekly cyclic component (i.e., freq=7)
startdate = as.numeric(covid19$date[1])
out = beast(sqrt(covid19$newcases), start= startdate, deltat=1, freq=7)
out$time = as.Date(out$time, origin='1970-01-01') # Convert from integers to Date.
plot( out, main='Changepoint analaysi for the full dataset')
###Introduce NAs at 200 random locations out of 694
set.seed(1234)
newcasesNA = covid19$newcases
newcasesNA[ sample(length(newcasesNA), 200) ]= NA
out1 = beast(sqrt(newcasesNA), start= startdate, deltat=1, freq=7)
out1$time = as.Date(out1$time, origin='1970-01-01') # Convert from integers to Date.
plot( out1, main='Changepoint analaysis with 200 missing datapoints')
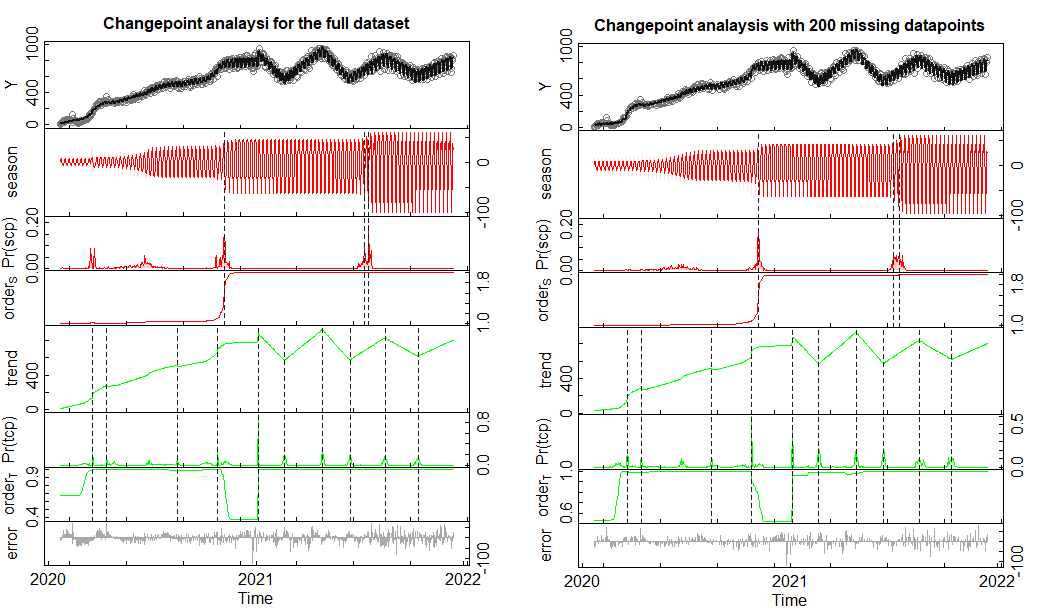
In the figure above, the time series was decomposed into two components: a periodic curve together with periodic structural changes (i.e., Pr(scp), and a trend curve together with trend changepoints (i.e., Pr(tcp)).