gamlss (Generalized Additive Models for Location Scale and Shape) can help you model any underlying nonlinearities while also accounting for the changes in distribution.
The original paper's abstract describes it well:
A general class of statistical models for a univariate response
variable is presented which we call the generalized additive model for
location, scale and shape (GAMLSS). The model assumes independent
observations of the response variable y given the parameters, the
explanatory variables and the values of the random effects. The
distribution for the response variable in the GAMLSS can be selected
from a very general family of distributions including highly skew or
kurtotic continuous and discrete distributions. The systematic part of
the model is expanded to allow modelling not only of the mean (or
location) but also of the other parameters of the distribution of y,
as parametric and/or additive nonparametric (smooth) functions of
explanatory variables and/or random-effects terms. Maximum (penalized)
likelihood estimation is used to fit the (non)parametric models. A
Newton–Raphson or Fisher scoring algorithm is used to maximize the
(penalized) likelihood. The additive terms in the model are fitted by
using a backfitting algorithm. Censored data are easily incorporated
into the framework. Five data sets from different fields of
application are analysed to emphasize the generality of the GAMLSS
class of models.
And its first figure illustrates its utility in your case:
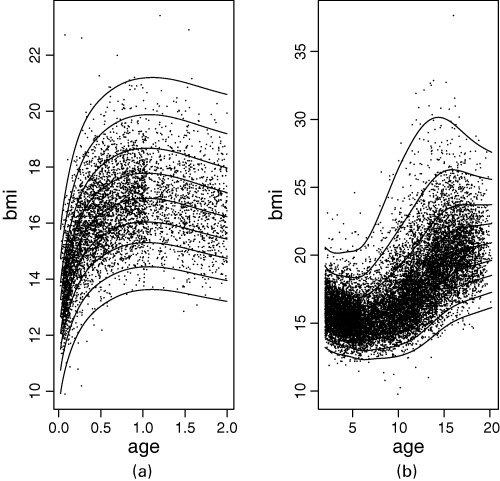
There is an R package by the same authors and a dedicated website that has tutorials such as this one.
Refs:
Rigby, R. A., & Stasinopoulos, D. M. (2005). Generalized additive models for location, scale and shape. Journal of the Royal Statistical Society Series C: Applied Statistics, 54(3), 507-554. https://doi.org/10.1111/j.1467-9876.2005.00510.x
Stasinopoulos, D. M., & Rigby, R. A. (2007). Generalized Additive Models for Location Scale and Shape (GAMLSS) in R. Journal of Statistical Software, 23(7), 1–46. https://doi.org/10.18637/jss.v023.i07