As you know, you look for patterns of (partial) autocorrelation coefficients that are large.
A rough guide to how large is "large" is to pay attention to any coefficient plotted with a spike extending beyond the two bands shown in the charts. (As produced by the stats:::acf.plot
function in R
, these bands enclose the middle 95% of a standard Normal distribution, implying that if the coefficients were independent -- which they are not -- then a white noise process would have, on average, 95% of its spikes inside the bands.)
In the original post, both the ACF and PACF exhibited four such large spikes apiece out of 36 (nonzero) lags, but none of the spikes went far beyond the bands; the longest was not even twice as far out. Since the expected number of large spikes is (100%-95%) times 36 = 1.8 per plot, maybe some of these large spikes mean something.
The question is, which of those spikes should you pay any attention to? Although a great deal can be said about this, let's first check to what extent those charts appear to offer any significant evidence of correlation at all. To do so, I generated 500 independent series of Gaussian white noise of length 5000, computed their ACF and PACF functions from lags 1 through 36, and counted how many spikes in each chart fell outside the bands. Here is one such pair of charts. Coincidentally, it exhibits four such spikes in each chart.
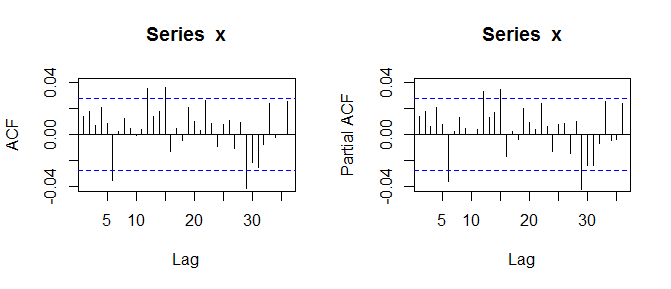
I will summarize all 500 of the simulated series with a scatterplot of those spike counts.
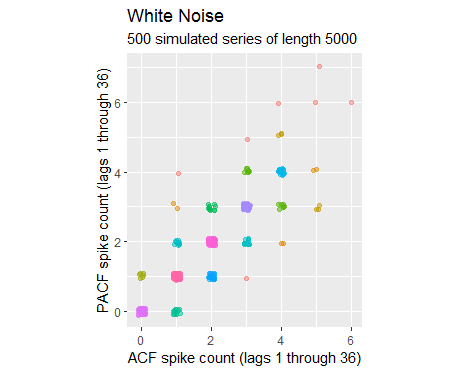
The points are jittered a little to resolve overlaps. The cluster of light blue dots around $(4,4)$ documents 21 series within the 500 that had four large spikes in each plot. The dots to its right and above document another 13 such series, for a total of 34, or 6.8% of them all. That's a little unusual but not rare. Consequently, we may conclude that four relatively small spikes in each plot does not especially distinguish the series from white noise.
Accordingly, because we have little evidence that any of these spikes means anything, you may elect to proceed as if you have captured all the significant correlation structure in the series through your differencing and removal of seasonality: there is no need to include an autoregressive or a moving average component.
As a double check, if there truly were such components they ought to exhibit themselves as consistently decaying spikes at the shortest lags--but that's not the case in your plots. And, if there were residual seasonality, it would be manifest in a regular series of noticeable spikes at constant intervals, such as every 3, 6, or 12 lags. That's not the case in your plots, either.