Your comments above lead me to believe that you are asking about the distribution of $X$ marginalized over the classes. This marginal distribution is Gaussian in the first dimension but not in the second.
Since the first and second dimensions are independent they can be treated separately. The means and variances conditional on class in the first dimension are the same for both classes, so mean and variance marginalized over class are the same those conditional on class $(\mu_1 = 1,\,\sigma_{1}^2 = 2)$. In the second dimension the marginal distribution is a mixture of Gaussians, which is not itself Gaussian:
$p(x_2) = \frac{1}{2}\text{N}(1,1) + \frac{1}{2}\text{N}(3,1),$
in which $\text{N}(\mu, \sigma^2)$ is the probability density of the Gaussian (aka normal) distribution with mean $\mu$ and variance $\sigma^2$.
The mean of the second dimension variable is
$\text{E}(X_2) = \Pr(C_1)\text{E}(X_2|C_1) + \Pr(C_2) \text{E}(X_2|C_2)$
$\text{E}(X_2) = \frac{1}{2} \cdot 1 + \frac{1}{2} \cdot 3 = 2.$
And now the variance. In general,
$\text{Var}(Y) = \text{E}(Y^2) - \left[\text{E}(Y)\right]^2.$
We'll find it useful to rearrange this as
$\text{E}(Y^2) = \text{Var}(Y) + \left[\text{E}(Y)\right]^2.$
So
$\text{E}(X_2^2) = \Pr(C_1)\text{E}(X_2^2|C_1) + \Pr(C_2) \text{E}(X_2^2|C_2)$
$\text{E}(X_2^2) = \frac{1}{2}(\text{Var}(X_2|C_1) + \left[\text{E}(X_2|C_1)\right]^2$
$+\text{Var}(X_2|C_2) + \left[\text{E}(X_2|C_2)\right]^2)$
$\text{E}(X_2^2) = \frac{1}{2}(1 + 1^2 + 1 + 3^2) = 6.$
Now we can get
$\text{Var}(X_2) = \text{E}(X_2^2) - \left[\text{E}(X_2)\right]^2 = 6 - 2^2 = 2.$
Here's what the distribution of $X_2$ looks like, along with a Gaussian distribution with the same mean and variance.
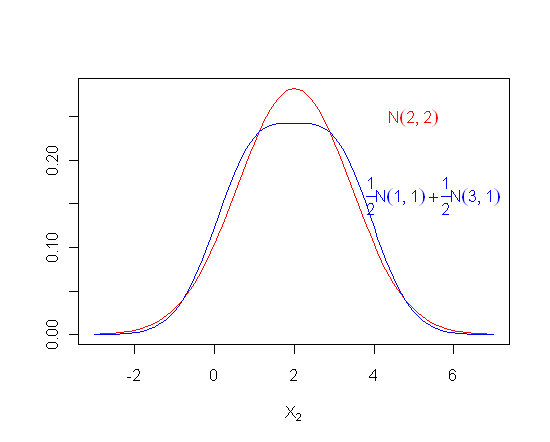