- What's the significance of the order of parameters separated by a semicolon, e.g. $(\theta;x)$ vs. $(x;\theta)$?
- Do $f(x;\theta)$ and $f(x|\theta)$ refer to the same function?
The function $f(\theta;x)$ and $f(x;\theta)$ are the same. It converts two inputs $x$ and $\theta$ into a number.
Below you see a surface plot that makes this visible. (from the question The basic logic of constructing a confidence interval)
The significance of the order in $(\theta;x)$ vs $(x;\theta)$ occurs when you take a 'slice' out of this 3d picture. This can be done in different directions
- When you have the slices in direction left to right, along the $x$ axis, the interpretation is as a probability density function (pdf)
- When you slice in the direction back to front, along the $\theta$ axis, then the interpretation is the likelihood function.
Note that in the image below the function is not the same as a joint probability density. Only the slices in one direction relate to a probability density. What is done in the image, is that we stack multiple probability density functions, by varying $\theta$, which is creating a 3d image out of multiple slices.
This likelihood function, should not be tried to be overinterpreted. It is not really a goal by itselve and more like a tool that is used for analysis. If you like then you could interpret likelihood function as a measure for how strong evidence a particular observation $x$ is for different values of the parameter $\theta$. It is a function of both $\theta$ and $x$ but in the application we often have $x$ fixed. We know the observation and want to use $x$ to infer something about $\theta$, which may be an unknown parameter. The Bayes factor relates to this interpretation.
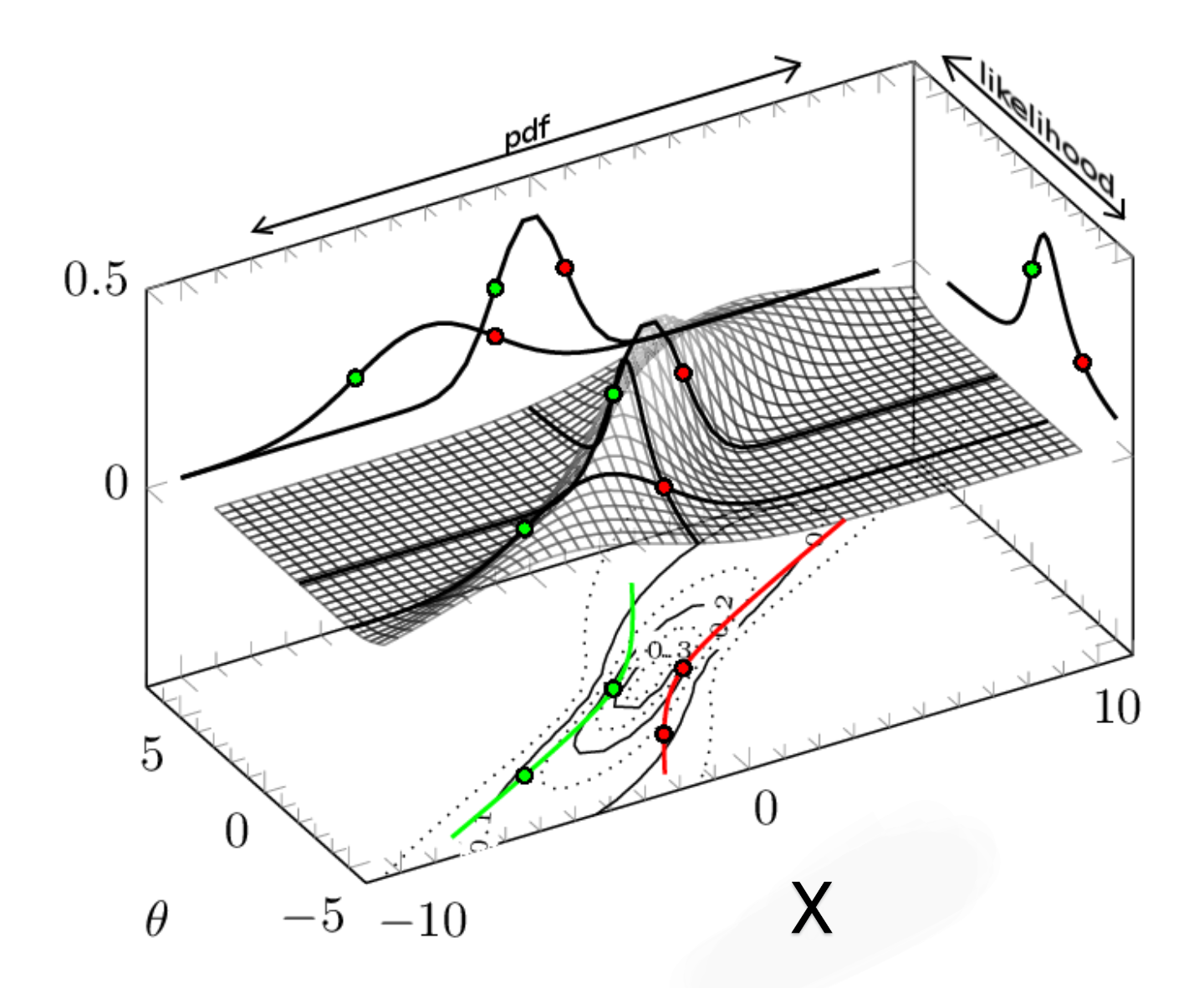
- $f(x|\theta)$ has a 'given' bar like a conditional but the third-year notes appear to refer to it as a 'joint'. Which is it? and why the apparent mis-match?
I can not make sense of the lecture notes $f(x|\theta) = a(x)g(\theta,x)$
- Is the likelihood $L$ equal to the other function $f$ or proportional? Is it proportional in general and sometimes equal as a special case, with a proportionality constant of $1$?
In your class they introduced the likelihood as being equal to the conditional probability $\mathcal{L}(\theta;x) = f(x;\theta)$, but this was just a simplification. The likelihood does not need to be equal and it is proportional. The reason that it does not need to be equal, is because in the practical application the ratio's of likelihood are used and any constant of proportionality will be eliminated. And also the reason that we want to define it this way (with the proportional I stead of equal) is because often we do not know the exact probability distribution but we do know it relatively (we might know the shape and proportions, but not the exact values).